I’ve always been excited about AI’s fast progress, especially in language models. They can handle complex tasks easily. But, I found something strange recently. It seems that bigger AIs get worse at answering simple questions
This goes against the idea that bigger is always better. It’s a mystery that has made me think a lot about these advanced systems.
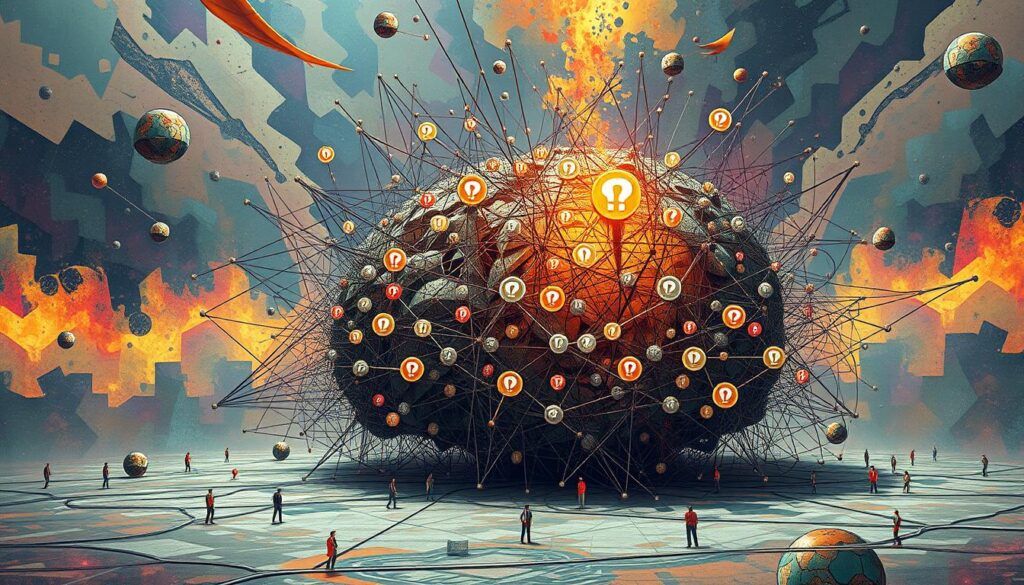
This problem shows the big challenges that researchers and developers face. They’re trying to make AI better, but it’s not easy. In this article, we’ll look into why bigger AI models might not always be better.
We’ll also talk about the limits of language models and the “hallucination problem.” It’s a complex issue that shows the unexpected challenges of these powerful technologies.
Understanding the Paradox of AI Model Scaling
The field of artificial intelligence is growing fast. Model scaling is now a big topic. It’s thought that bigger language models mean better performance and accuracy. But, the truth is more complicated.
Looking into how model size affects performance shows a paradox. This paradox questions the simple idea that bigger models are always better.
Exploring the Relationship Between Model Size and Performance
Bigger language models are seen as the answer to better accuracy and skills. But, the link between model size and performance isn’t straightforward. Studies show that as models get bigger, the extra benefits in performance start to fade or stop growing.
Challenges in Maintaining Accuracy with Larger Models
As language models get more complex, keeping accuracy and performance is harder. The huge amount of data and the complexity of model scaling cause problems. These include overfitting, less generalization, and odd behaviors.
Dealing with these issues needs a better grasp of what affects model performance.
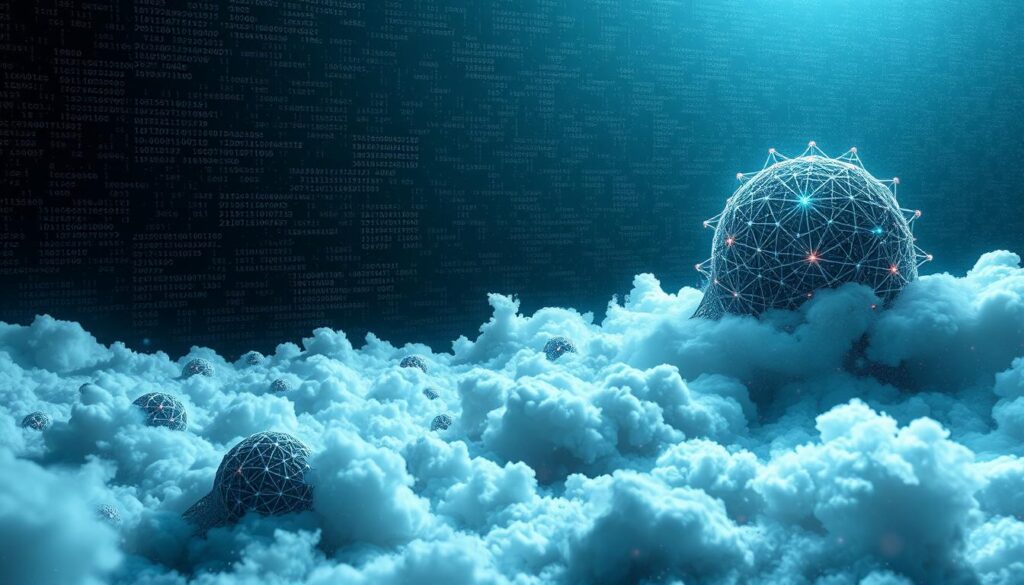
The Limitations of Language Models in Question-Answering
Artificial intelligence has made big strides, and language models are now key for answering questions. Yet, they face big challenges, especially in giving correct and honest answers to simple questions.
Language models struggle with understanding and reasoning. They are trained on a lot of data but don’t always get the world right. This can cause them to make up answers that seem right but aren’t.
They also have trouble understanding the context of questions. This can lead to answers that don’t match what was asked or are not relevant.
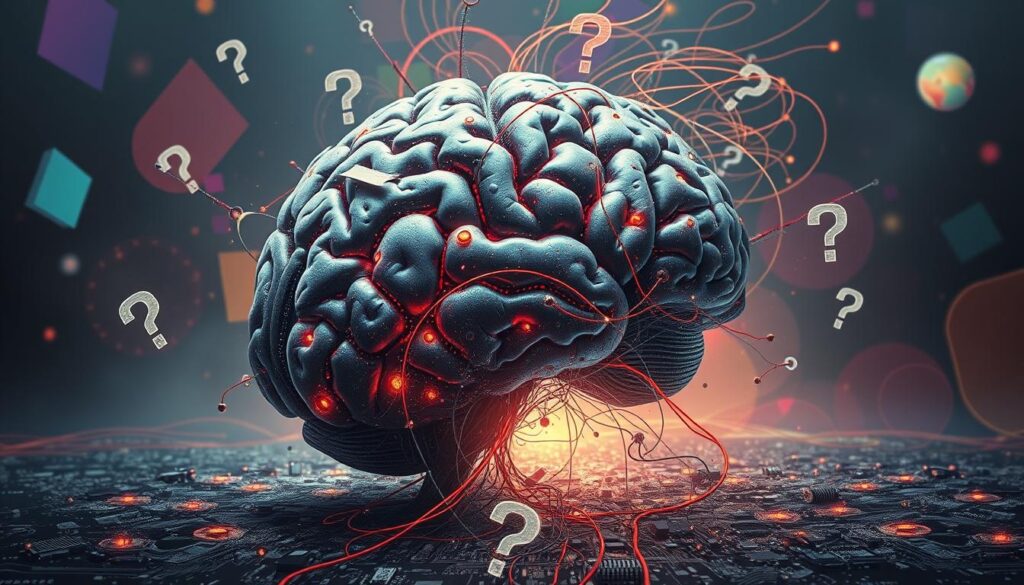
Moreover, language models can’t always do complex thinking. They might not make connections between different pieces of information. This is a big problem for questions that need deep understanding.
Even so, language models are getting better at answering questions. But, it’s important to remember their limits. We should always check their answers carefully, especially when we need accurate information.
AIs Get Worse at Answering Simple Questions as They Get Bigger
As AI models grow, a surprising issue has come up. Larger models often do worse on simple questions than smaller ones. This has been seen through empirical evidence and case studies. It shows how AI model scaling affects performance.
Empirical Evidence and Case Studies
Recent studies have shown that big AI models struggle with simple questions. For instance, a study by a top AI lab found a large model couldn’t answer basic questions right. It gave weird or opposite answers, even though it did well on harder tasks. Case studies from experts also point to the same problem. They say bigger models can lose accuracy and reliability.
This shows that the link between AI model size and performance is complex. Just because an AI is big doesn’t mean it’s better at answering simple questions. We need to understand and fix this problem as AIs get worse at answering simple questions as they get bigger.
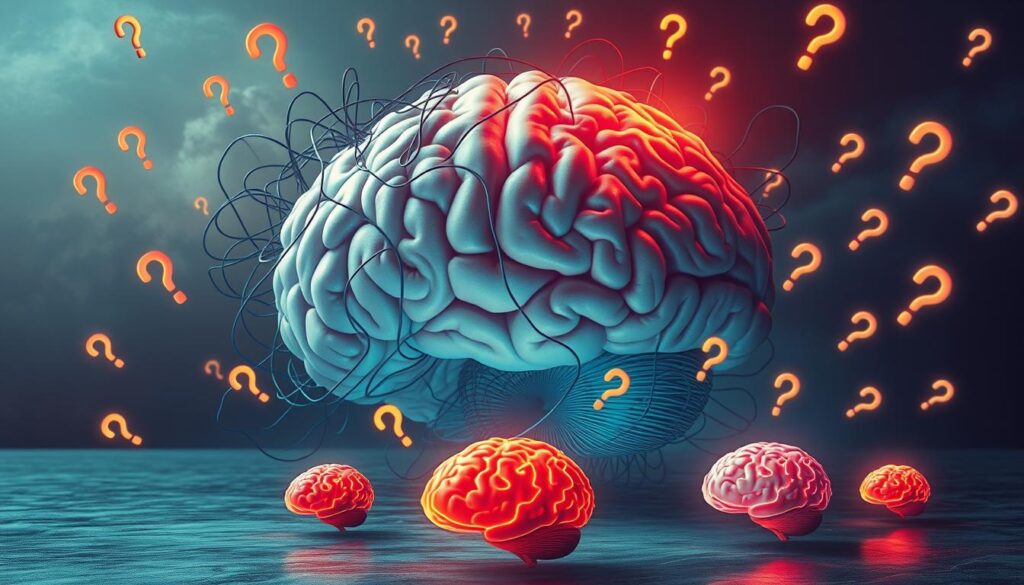
The Hallucination Problem in Large AI Models
AI models are getting bigger and more complex. But, they have a big problem – hallucination. This happens when they make up responses that sound real but are actually wrong. The reasons for this are many, including knowledge gaps, limited reasoning, and a tendency to create convincing but false answers.
Causes and Consequences of Hallucination
Large AI models often can’t understand the information they’ve been trained on well. They might not connect different pieces of knowledge right. This leads to answers that seem right but aren’t. Also, the huge amount of data they’re trained on can leave gaps in their knowledge.
The effects of hallucination in AI models are serious. It can make people doubt the accuracy of these systems. False information from AI can lead to bad decisions and harm trust in technology. So, fixing the hallucination problem is key for AI’s future, especially in important areas like healthcare and finance.